views
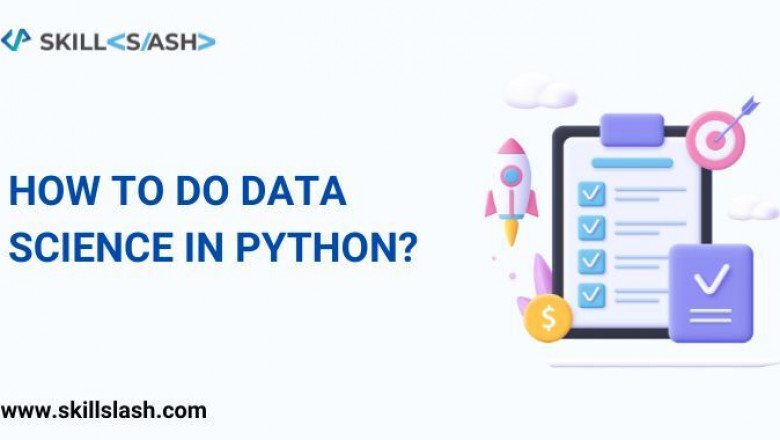
How to do data science in Python?
Python has become the go-to language for data science, and there are many reasons for this. It is easy to learn and use, it has a wide range of libraries for data analysis and manipulation, and integrates well with other programming languages. In this article, we will explore how to do data science in Python.
What is data science?
Data science is an interdisciplinary field that uses scientific methods, processes, algorithms, and systems to extract knowledge and insights from data in various forms, both structured and unstructured, similar to data mining.
Data science is a concept to unify statistics, data analysis, machine learning, and their related methods to understand and analyze actual phenomena with data. It employs techniques and theories drawn from many fields within the broad areas of mathematics, statistics, information science, and computer science. In this way, data science represents a merging of prior disciplines, where the goal is to enhance predictive analytics, machine learning, and artificial intelligence by finding hidden patterns or latent structures in data sets.
What is Python?
Python is a high-level, interpreted, general-purpose programming language, created on December 3, 1989, by Guido van Rossum, with a design philosophy entitled, "There's only one way to do it, and that's why it works." An open-source programming language, its syntax is very easy to learn and has high readability.
Python's large support libraries, user-friendliness, and rich, built-in data structures make it a popular choice. Additionally, Python's optional dynamic high-level data type shortens the necessary length of support code. Python's clear object-oriented design facilitates better process management.
What are the benefits of doing data science in Python?
Python is an unambiguous, easy-to-read, general-purpose high-level programming language that considers paradigms of structured, procedural, and object-oriented programming.
● Better readability and writing
With a philosophy that emphasizes code readability, Python aims to help programmers write clear, logical code for small and large-scale projects.
● Better integrations
In addition to offering standard features like those found in other widely used languages, Python’s standard library provides tools for data science that can be integrated into existing workflows or used to start new projects from scratch.
● Simple syntax
Python’s simple syntax rules allow for ease of use and debugging while its large selection of modules makes it applicable to many different tasks.
● Comprehensibility
Some other benefits of doing data science in Python are that Python can be used for prototyping and is also popular for software development due to its comprehensibility.
● A stack of libraries
Additionally, the Python Package Index (PyPI) has a wealth of libraries available which extend the capabilities of the language even further. Not only does this make starting new projects easier but it can also save time as certain tasks do not need to be coded from scratch.
How to get started with data science in Python?
Python is a powerful programming language that is widely used in many industries today. Python is easy to learn for beginners and has many modules and libraries that allow for robust data analysis.
● Anaconda: There are many ways to get started with data science in Python. One way is to start by installing the Anaconda distribution, which comes with all the necessary tools for data science, including Jupyter Notebooks, NumPy, pandas, matplotlib, and scikit-learn.
● Jupyter Notebooks: Once you have Anaconda installed, you can launch Jupyter Notebooks from the Anaconda Navigator program. Jupyter Notebooks are an interactive coding environment that allow you to write and execute code in Python (and other languages).
● Popular platforms: Another way to get started with data science in Python is to use a platform such as Kaggle or Google Colaboratory. Kaggle is a website that hosts data science competitions, while Google Colaboratory is a free online coding environment that allows you to write and execute code in Python (and other languages).
● Working with data sets: Once you have chosen your preferred environment, you can start working with datasets. The NumPy library offers several functions for loading and manipulating numerical data, while the pandas library provides functions for working with tabular data. matplotlib and seaborn are two popular libraries for visualizing data in Python. scikit-learn is a library that provides implementations of many popular machine learning algorithms. The barrier to entry for writing machine learning models is greatly reduced by adhering to a clear, consistent code style, which guarantees that your machine learning code is straightforward to comprehend and reproduce.
Conclusion
Data science is a rapidly growing field, and Python is a popular language for data science. If you're interested in learning how to do data science in Python, there are many resources available to help you get started. In this article, we've given you an overview of some of the most popular resources for learning data science in Python. We hope that this has helped you get started on your journey to becoming a data scientist.
If you want to kickstart your journey in data science and master Python and other core technical concepts, Skillslash is the best support system you can find to get real-world experience and the skill set to thrive in this domain. With its Data Science Course In Delhi with placement guarantee, you can learn from industry experts, work with top AI firms, and receive unlimited job referral. Skillslash can help you get into it with its Data science course in Nagpur. Get in touch with the support team to know more.