views
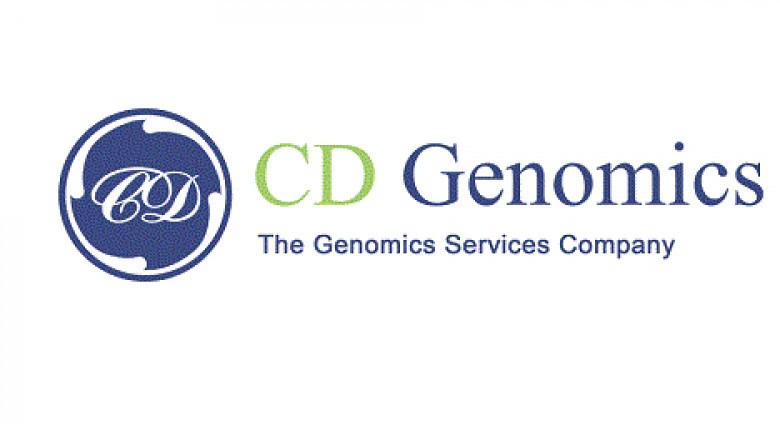
Viral metagenomics is the study of viruses in environmental and biological samples by utilizing next generation sequencing that generates very large data sets. Viral metagenomics analyzes viral sequences to deduce the impact of viruses on the environment of human health. Unlike amplicon sequencing, metagenomics obtains and investigates genetic material directly from environmental samples, which has led to a new understanding of the diversity and function of the microbial world. Bioinformatics analysis is one of the most important procedures for this purpose.
Bioinformatics pipeline for viral metagenomics
The general bioinformatics pipeline for viral metagenomics includes quality checking and filtering, assembly, as well as taxonomic classification and binning. There are two types of methods for taxonomic classification, i.e., similarity-based methods and composition-based methods. One representative example of similarity-based taxonomic classification is the NCBI BLAST searches. Taxonomic classification methods that explore composition of genome such as GC content, or short oligomer (k-mers) usage are known as composition-based methods, which can be used for taxonomic classification of sequences that do not have any homologs or are highly divergent from sequences in databases. Compared with similarity-based methods, composition-based methods have lower accuracy and are largely dependent on sequence length.
VIROME
The Viral Informatics Resource for Metagenome Exploration (VIROME), first described by Wommack et al. (2012), emphasizes the classification of viral metagenome sequencing (predicted open reading frames, ORFs) based on homology analysis. The VIROME analysis relies on three subject protein sequence databases, five annotated databases (SEED, ACLAME, COG, GO, and KEGG), the UniVec database, and CD-Hit 454. The CD-Hit 454 algorithm is used to search sequence libraries from the 454 pyrosequencer for false duplicate sequences. The UniRef 100 peptide database is used to detect viral metagenome sequences with similarity to known proteins. The MetaGenomes On-line (MGOL) peptide database contains predicted peptide sequences from 137 metagenome libraries, which is used for detection of similarity to unknown environmental sequences.
The VIROME pipeline involves quality control, sequence analysis, functional and taxonomic ORF characterization, ORF classification, and environmental characterization. Each sequence is first trimmed for quality and trimmed of linker, adapter, barcode sequencer, and probably false duplicate reads. Subsequently, sequencing reads that have significant homology to a ribosomal RNA (rRNA) sequence are removed using BLASTN against rRNA subject database. tRNAscan-SE are used to screen the presence of tRNAs and ORFs using MetaGene Annotator. A multi-fasta file of peptide sequences is then constructed and analyzed using BLASTP against the UniRef 100 and MGOL databases. Predicted peptides can also be characterized using the annotated sequence databases. Based on the results of BLASTP analyses, each predicted viral metagenome peptide is divided into seven VIROME classes.
Metavir 2
Metavir 2, described by Roux et al. (2014), is the first tools designed for a comprehensive analysis of assembled virome sequences. Metavir is dedicated to the analysis of viromes uploaded by registers, either virome composed of raw reads or viromes assembled into contigs. Many softwares are available for the assembly step: Newbler can be used for 454 data, and Illumina data can be assembled using SOAP, MetaVelvet, OptiDBA, and Idba-ud.
For unassembled reads
Virome reads are first compared to the complete viral genomes of the RefSeq Virus database using BLAST, in order to determine taxonomic composition. K–mer frequency distribution bias is computed for all datasets without size restriction. Phylogenetic analyses are computed with FastTree. Based on the BLAST hit results against RefseqVirus, two types of recruitment plots can be made: a scatter plot and a histogram.
For assembled viromes
For assembled viromes, ORFs are first predicted through MetaGeneAnnotator. All predicted translated ORFs are then compared to databases including RefseqVirus protein database using BLASTp, and PFAM database of protein domains using HMMScan.
An interactive genomic map can be made for contig display by using RaphaelSVG and Raphael-zpd plugin. Similarities between contigs and between contigs and viral genomes can be visualized as an interactive network created with Cytoscape-web. Associated with this network, the collinearity between contigs and genomes or other contigs can be displayed through RaphaelSVG and Raphael-zpd.